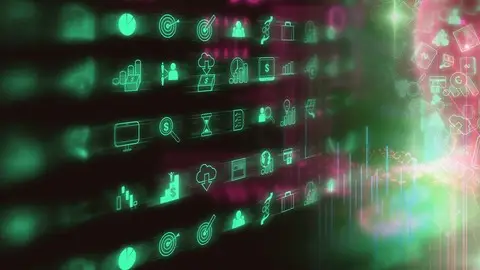
Recommender Systems And Deep Learning In Python
Last updated 10/2022
MP4 | Video: h264, 1280x720 | Audio: AAC, 44.1 KHz
Language: English
| Duration: 12h 31m
The most in-depth course on recommendation systems with deep learning, machine learning, data science, and AI techniques
What you'll learn
Understand and implement accurate recommendations for your users using simple and state-of-the-art algorithms
Big data matrix factorization on Spark with an AWS EC2 cluster
Matrix factorization / SVD in pure Numpy
Matrix factorization in Keras
Deep neural networks, residual networks, and autoencoder in Keras
Restricted Boltzmann Machine in Tensorflow
Requirements
For earlier sections, just know some basic arithmetic
For advanced sections, know calculus, linear algebra, and probability for a deeper understanding
Be proficient in Python and the Numpy stack (see my free course)
For the deep learning section, know the basics of using Keras
Description
Believe it or not, almost all online businesses today make use of recommender systems in some way or another.What do I mean by "recommender systems", and why are they useful?Let's look at the top 3 websites on the Internet, according to Alexa: Google, YouTube, and Facebook.Recommender systems form the very foundation of these technologies.Google: Search resultsThey are why Google is the most successful technology company today.YouTube: Video dashboardI'm sure I'm not the only one who's accidentally spent hours on YouTube when I had more important things to do! Just how do they convince you to do that?That's right. Recommender systems!Facebook: So powerful that world governments are worried that the newsfeed has too much influence on people! (Or maybe they are worried about losing their own power. hmm.)Amazing!This course is a big bag of tricks that make recommender systems work across multiple platforms.We'll look at popular news feed algorithms, like Reddit, Hacker News, and Google PageRank.We'll look at Bayesian recommendation techniques that are being used by a large number of media companies today.But this course isn't just about news feeds.Companies like Amazon, Netflix, and Spotify have been using recommendations to suggest products, movies, and music to customers for many years now.These algorithms have led to billions of dollars in added revenue.So I assure you, what you're about to learn in this course is very real, very applicable, and will have a huge impact on your business.For those of you who like to dig deep into the theory to understand how things really work, you know this is my specialty and there will be no shortage of that in this course. We'll be covering state of the art algorithms like matrix factorization and deep learning (making use of both supervised and unsupervised learning - Autoencoders and Restricted Boltzmann Machines), and you'll learn a bag full of tricks to improve upon baseline results.As a bonus, we will also look how to perform matrix factorization using big data in Spark. We will create a cluster using Amazon EC2 instances with Amazon Web Services (AWS). Most other courses and tutorials look at the MovieLens 100k dataset - that is puny! Our examples make use of MovieLens 20 million.Whether you sell products in your e-commerce store, or you simply write a blog - you can use these techniques to show the right recommendations to your users at the right time.If you're an employee at a company, you can use these techniques to impress your manager and get a raise!I'll see you in class!NOTE:This course is not "officially" part of my deep learning series. It contains a strong deep learning component, but there are many concepts in the course that are totally unrelated to deep learning."If you can't implement it, you don't understand it"Or as the great physicist Richard Feynman said: "What I cannot create, I do not understand".My courses are the ONLY courses where you will learn how to implement machine learning algorithms from scratchOther courses will teach you how to plug in your data into a library, but do you really need help with 3 lines of code?After doing the same thing with 10 datasets, you realize you didn't learn 10 things. You learned 1 thing, and just repeated the same 3 lines of code 10 times.Suggested Prerequisites:For earlier sections, just know some basic arithmeticFor advanced sections, know calculus, linear algebra, and probability for a deeper understandingBe proficient in Python and the Numpy stack (see my free course)For the deep learning section, know the basics of using KerasFor the RBM section, know TensorflowWHAT ORDER SHOULD I TAKE YOUR COURSES IN?:Check out the lecture "Machine Learning and AI Prerequisite Roadmap" (available in the FAQ of any of my courses, including the free Numpy course)
Overview
Section 1: Welcome
Lecture 1 Introduction
Lecture 2 Outline of the course
Lecture 3 Where to get the code
Lecture 4 How to Succeed in this Course
Section 2: Simple Recommendation Systems
Lecture 5 Section Introduction and Outline
Lecture 6 Perspective for this Section
Lecture 7 Basic Intuitions
Lecture 8 Associations
Lecture 9 Hacker News - Will you be penalized for talking about the NSA?
Lecture 10 Reddit - Should censorship based on politics be allowed?
Lecture 11 Problems with Average Rating & Explore vs. Exploit (part 1)
Lecture 12 Problems with Average Rating & Explore vs. Exploit (part 2)
Lecture 13 Bayesian Ranking (Beginner Version)
Lecture 14 Demographics and Supervised Learning
Lecture 15 PageRank (part 1)
Lecture 16 PageRank (part 2)
Lecture 17 Evaluating a Ranking
Lecture 18 Section Conclusion
Lecture 19 Suggestion Box
Section 3: Collaborative Filtering
Lecture 20 Collaborative Filtering Section Introduction
Lecture 21 User-User Collaborative Filtering
Lecture 22 Collaborative Filtering Exercise Prep
Lecture 23 Data Preprocessing
Lecture 24 User-User Collaborative Filtering in Code
Lecture 25 Item-Item Collaborative Filtering
Lecture 26 Item-Item Collaborative Filtering in Code
Lecture 27 Collaborative Filtering Section Conclusion
Section 4: Beginner Q&A
Lecture 28 How do I Choose Which Model to Use?
Lecture 29 How do I Solve the Cold-Start Problem?
Lecture 30 What if I Don't Like Math or Programming?
Section 5: Matrix Factorization and Deep Learning
Lecture 31 Matrix Factorization Section Introduction
Lecture 32 Matrix Factorization - First Steps
Lecture 33 Matrix Factorization - Training
Lecture 34 Matrix Factorization - Expanding Our Model
Lecture 35 Matrix Factorization - Regularization
Lecture 36 Matrix Factorization - Exercise Prompt
Lecture 37 Matrix Factorization in Code
Lecture 38 Matrix Factorization in Code - Vectorized
Lecture 39 SVD (Singular Value Decomposition)
Lecture 40 Probabilistic Matrix Factorization
Lecture 41 Bayesian Matrix Factorization
Lecture 42 Matrix Factorization in Keras (Discussion)
Lecture 43 Matrix Factorization in Keras (Code)
Lecture 44 Deep Neural Network (Discussion)
Lecture 45 Deep Neural Network (Code)
Lecture 46 Residual Learning (Discussion)
Lecture 47 Residual Learning (Code)
Lecture 48 Autoencoders (AutoRec) Discussion
Lecture 49 Autoencoders (AutoRec) Code
Section 6: Restricted Boltzmann Machines (RBMs) for Collaborative Filtering
Lecture 50 RBMs for Collaborative Filtering Section Introduction
Lecture 51 Intro to RBMs
Lecture 52 Motivation Behind RBMs
Lecture 53 Intractability
Lecture 54 Neural Network Equations
Lecture 55 Training an RBM (part 1)
Lecture 56 Training an RBM (part 2)
Lecture 57 Training an RBM (part 3) - Free Energy
Lecture 58 Categorical RBM for Recommender System Ratings
Lecture 59 RBM Code pt 1
Lecture 60 RBM Code pt 2
Lecture 61 RBM Code pt 3
Lecture 62 Speeding up the RBM Code
Section 7: Big Data Matrix Factorization with Spark Cluster on AWS / EC2
Lecture 63 Big Data and Spark Section Introduction
Lecture 64 Setting up Spark in your Local Environment
Lecture 65 Matrix Factorization in Spark
Lecture 66 Spark Submit
Lecture 67 Setting up a Spark Cluster on AWS / EC2
Lecture 68 Making Predictions in the Real World
Section 8: Basics Review
Lecture 69 (Review) Keras Discussion
Lecture 70 (Review) Keras Neural Network in Code
Lecture 71 (Review) Keras Functional API
Lecture 72 (Review) How to easily convert Keras into Tensorflow 2.0 code
Lecture 73 (Review) Confidence Intervals
Lecture 74 (Review) Gaussian Conjugate Prior
Section 9: Bayesian Ranking (Scary Version)
Lecture 75 Bayesian Approach part 0 (Preparation)
Lecture 76 Bayesian Approach part 1 (Optional)
Lecture 77 Optional: Bayesian Approach part 2 (Sampling and Ranking)
Lecture 78 Optional: Bayesian Approach part 3 (Gaussian)
Lecture 79 Optional: Bayesian Approach part 4 (Code)
Lecture 80 Why don't we just use a library?
Section 10: Setting Up Your Environment (FAQ by Student Request)
Lecture 81 Anaconda Environment Setup
Lecture 82 How to How to install Numpy, Theano, Tensorflow, etc.
Section 11: Extra Help With Python Coding for Beginners (FAQ by Student Request)
Lecture 83 How to Code by Yourself (part 1)
Lecture 84 How to Code by Yourself (part 2)
Lecture 85 Proof that using Jupyter Notebook is the same as not using it
Lecture 86 Python 2 vs Python 3
Section 12: Effective Learning Strategies for Machine Learning (FAQ by Student Request)
Lecture 87 How to Succeed in this Course (Long Version)
Lecture 88 Is this for Beginners or Experts? Academic or Practical? Fast or slow-paced?
Lecture 89 Machine Learning and AI Prerequisite Roadmap (pt 1)
Lecture 90 Machine Learning and AI Prerequisite Roadmap (pt 2)
Section 13: Appendix / FAQ Finale
Lecture 91 What is the Appendix?
Lecture 92 BONUS
Anyone who owns or operates an Internet business,Students in machine learning, deep learning, artificial intelligence, and data science,Professionals in machine learning, deep learning, artificial intelligence, and data science
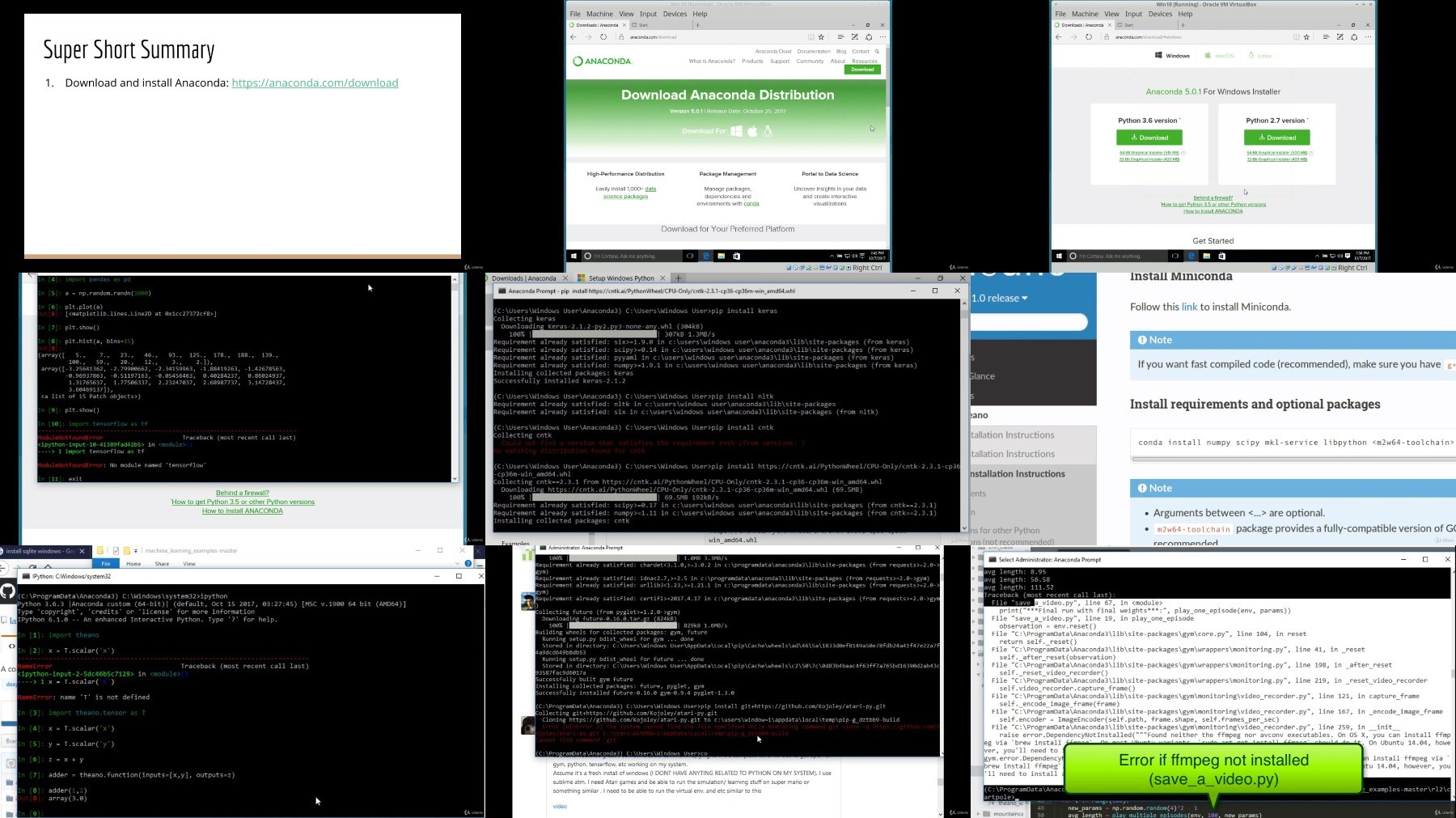
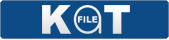
Free search engine download: Udemy Recommender Systems and Deep Learning in Python 2022 10